New Delhi: Scientists struggling with the manual interpretation of growing seismic data to explore causes of earthquake, particularly when the area is geologically complex, are now armed with a machine learning-based solution that can help in automatic interpretation of this data.
Effective detection of subsurface geologic features from surface seismic data is very important for understanding the geotectonic, basin evolution, resource exploration, and process that causes earthquakes (seismogenesis) of an area. For this, acquisition of seismic data keeps on growing, making the processing computationally intensive and interpretation tedious. Thanks to high-performance computing systems, that have allowed analysis of such voluminous data within a reasonable time after receiving guidance and inputs from interpreters. However, human analysts struggle for manual interpretation, particularly when the area is geologically complex and data is copious.
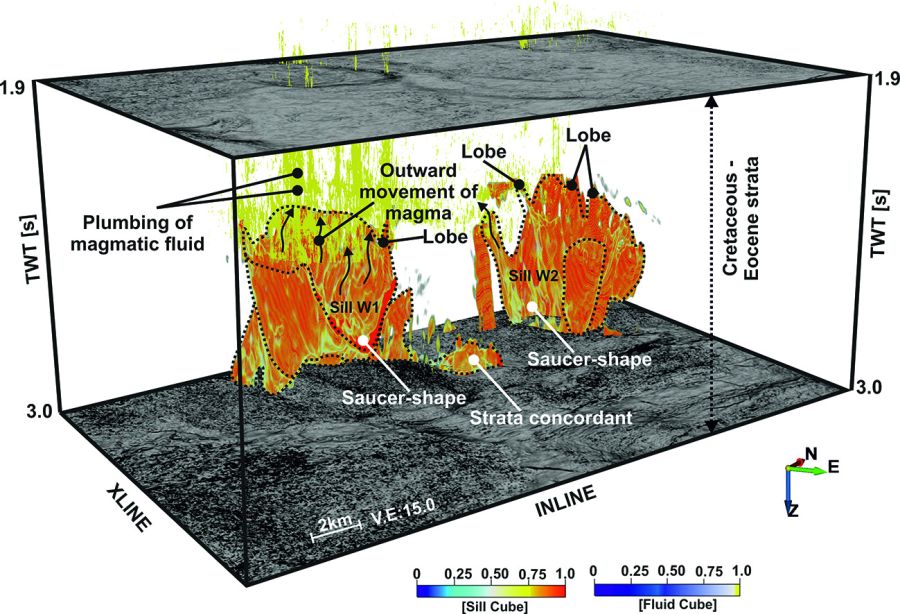
To automate the process and accelerate the interpretation, Scientists from Wadia Institute of Himalayan Geology (WIHG), an autonomous Institute under the Department of Science & Technology, Govt. of India, have developed a neural-based (machine learning-based) practical approach for automatic interpretation of 3D seismic data. This first of its kind approach has been developed by computing a new attribute called meta-attribute.
The tabular sheet intrusions between older layers of sedimentary rock or beds of volcanic lava (sill complexes) significantly contribute to the transport and storage of hot magma, and leading to overburden. This acts as plausible structural traps for hydrocarbon accumulations in sedimentary basin. The petroliferous (petroleum containing) Canterbury basin off New Zealand is a classic example, where saucer-shaped magmatic sills are embedded within the Cretaceous to Eocene (geological period that lasted from about 145 to 33.9 million years ago) succession resulting into forced folds and hydrothermal vents above the sill terminations.
Scientists from WIHG captured this scenario by designing a workflow and computing Sill Cube (SC) and Fluid Cube (FC) meta-attributes. These are hybrid attributes that are generated by amalgamating a number of seismic attributes (associated with the geologic targets) using a neural-based approach. This study is published in the research journal ‘Tectonophysics’. The WIHG team prepared the meta-attributes following supervised neural learning (machine learning), where computing systems are trained under the guidance of a human analyst.
According to the findings of this study, individual sills cover from 1.5 km2 to17 km2 areas, respectively. Moreover, the fluxed-out magmatic fluids from the tip of the principal sills vertically rise to a height of around 800 m through hydrothermal vents and uplift the overburden. Such an interpretational approach is automated and effectively narrates subsurface magmatic activities from 3D seismic data.
This work is an important step forward towards application of machine learning to address geological problems and looks promising in understanding complex geological processes in an active mountain belt such as the Himalaya.
